The advent of Artificial Intelligence (AI) has spurred a revolution across various industries, unleashing endless possibilities for innovation and efficiency.
AI now plays an integral role in everything from finance to healthcare, manufacturing to marketing, content creation to image generation, and much more. But it’s not a one-size-fits-all technology; there are different types – namely Generative AI and Predictive AI.
Two siblings born from the same mother but with wildly different talents. One predicts future trends with uncanny accuracy; the other dreams up things we humans haven’t even imagined yet.
Intrigued? You should be. Understanding these twins can help businesses leapfrog competition, skyrocket profits, and shape futures in unimaginable ways.
Rise of Artificial Intelligence
Artificial intelligence is fundamentally changing how we work, live, and play. According to Statista’s report on worldwide artificial intelligence revenues, global revenues have skyrocketed from $5 billion in 2015 to projected figures of over $125 billion by 2025.
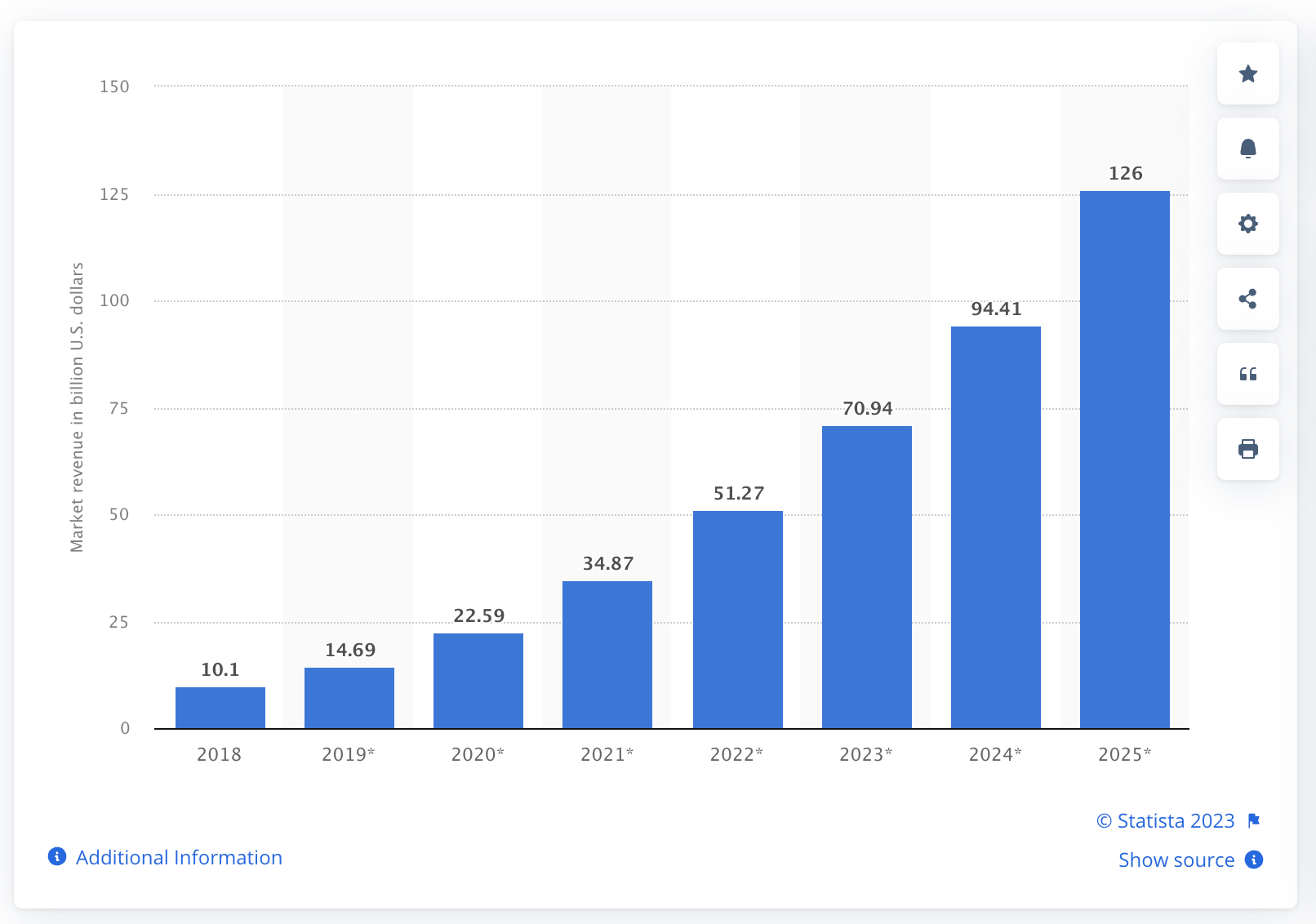
Generative AI vs Predictive AI
While both are rooted in the realm of artificial intelligence, each serves a distinct purpose.
Generative AI focuses on creating new data or content, often simulating human creativity, such as generating images or composing music.
In contrast, Predictive AI is all about forecasting future outcomes based on historical data, like predicting stock market trends or consumer behavior.
While Generative AI can be likened to an artist crafting a new masterpiece, Predictive AI is the strategist, using past events to anticipate the future. Together, they represent the diverse capabilities of AI, each with its unique applications and benefits to machine learning.
What is Generative AI?
If you’ve ever been amazed by a machine composing music or creating realistic images, then you have experienced the power of generative AI. But what exactly is it? Let’s get to the heart of it.
In a nutshell, generative AI employs algorithms and probabilistic models to fabricate something novel from pre-existing data. It can be thought of as an artist with its paintbrush – learning from numerous art pieces and then painting its own masterpiece.
The Nuts and Bolts
Generative AI relies on specific types of neural networks known as Generative Adversarial Networks (GANs). This technology involves two key components: a generator that creates new data instances, and a discriminator that evaluates them for authenticity. They work in tandem like competitive siblings; while one tries to produce real-looking fake content by mimicking human creativity, the other judges how well it has done so.
This push-and-pull dynamic allows GANs to improve over time through iterative training cycles, ultimately producing more refined outputs – whether they are text strings or complex 3D renderings.
A Palette Full Of Applications
The applications for generative AI are diverse – spanning across industries such as marketing, entertainment, healthcare & more. For instance, MuseNet, developed by OpenAI generates original songs encompassing multiple styles just based on brief musical prompts given.
Another interesting application is in image synthesis. This technology can generate new images or modify existing ones, creating everything from surreal landscapes to realistic human faces.
In the realm of marketing, generative AI applications can craft compelling copy that grabs attention and drives engagement. They learn from past campaigns and user behavior to deliver content tailored to your audience’s tastes.
It’s Not Just About Creativity
Though AI shines in the creativity department, it’s not all it can do. While generative AI’s creative prowess is evident, its practical applications are equally noteworthy. Beyond art and design, it plays a pivotal role in data augmentation, especially in sectors where data is scarce.
Generating synthetic data, aids in training robust machine learning models, ensuring they’re well-equipped even when real-world data is limited. Moreover, in research, generative AI can simulate complex scenarios or predict outcomes, providing insights without the need for costly real-world experiments.
In essence, generative AI is not just an artist; it’s a versatile tool that bridges creativity with practical solutions, pushing the boundaries of what’s possible.
Benefits of Using Generative AI
Generative AI, with its ability to create and innovate, offers businesses a unique advantage. From cost savings to enhanced personalization and smarter decision-making, the benefits of integrating generative AI into business operations are manifold. Let’s delve into some of these transformative advantages of machine learning.
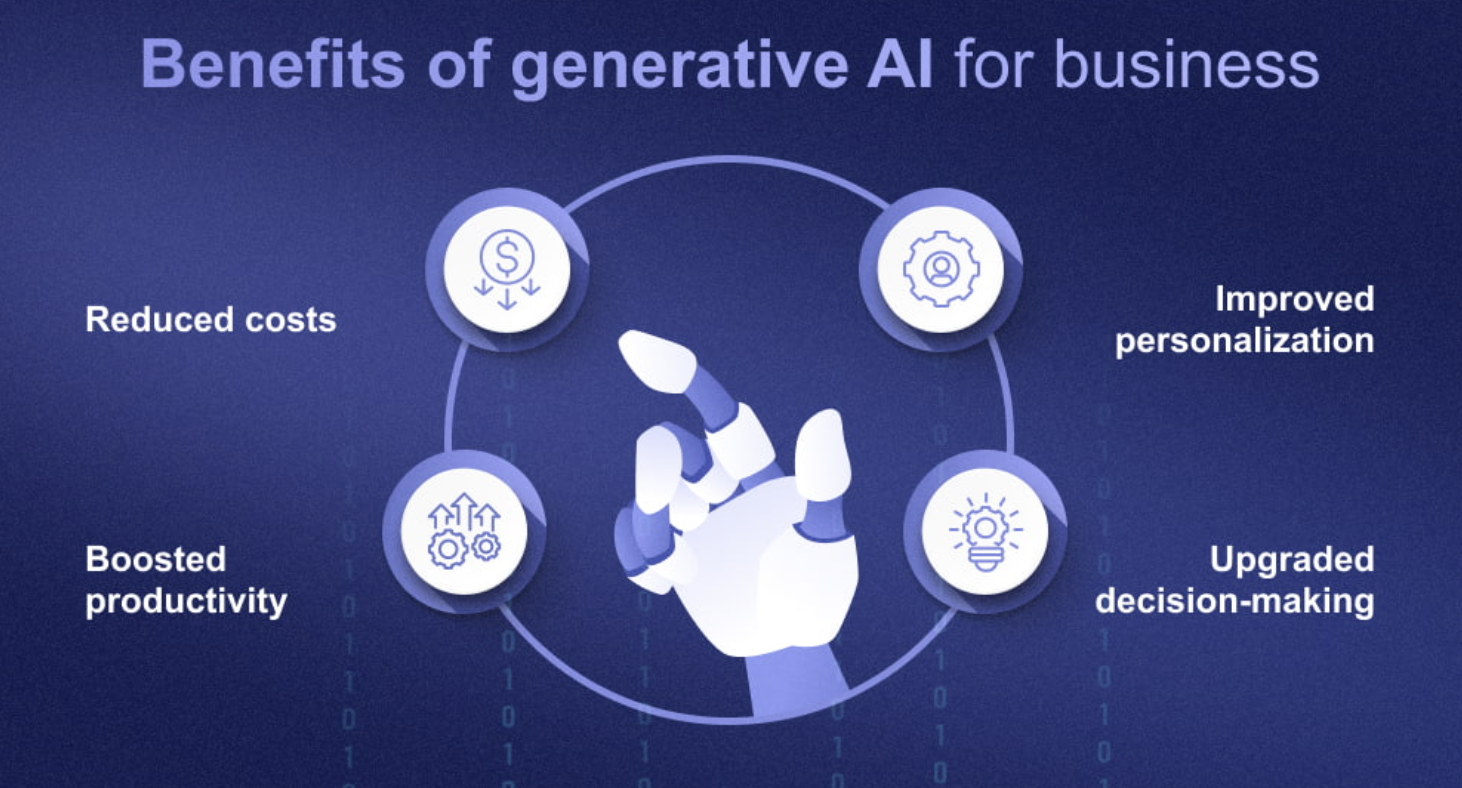
- Reduced Costs: One significant advantage of using generative AI is cost reduction. By automating routine tasks that would otherwise require human labor, companies can significantly cut down on operational expenses while maintaining or even improving efficiency levels.
- Increased Productivity: The automation capabilities provided by generative AI also lead to enhanced productivity. With less time spent on mundane tasks, employees can focus more on strategic activities that drive business growth and innovation. This results in higher output without necessarily increasing work hours or manpower.
- Improved Personalization: In today’s digital age where consumers demand personalized experiences, personalized marketing strategies, powered by generative AI, are a game-changer for businesses seeking to stand out from their competitors. The technology allows for better customer segmentation and targeting based on individual preferences and behaviors, thus delivering highly relevant content to each user.
- Improved Decision-Making: Last but not least is the impact of generative AI on decision-making processes within organizations. AI-driven decision-making not only speeds up the process but also enhances accuracy. The technology can analyze vast amounts of data and generate insights that help us make informed business decisions.
In essence, generative AI is a powerful tool for modern businesses seeking to stay competitive in an increasingly digital world. It’s high time companies explore this technology and leverage its potential benefits.
Key Thought:
Think of Generative AI as an artist wielding a brush, using complex algorithms and stats to craft new things from what’s already there. It leans on ever-evolving Generative Adversarial Networks (GANs) to refine its outputs – whether that’s text or intricate 3D renderings. From marketing to entertainment, this form of AI has wide-ranging applications.
What is Predictive AI?
Predictive AI, or predictive artificial intelligence, takes the guesswork out of decision-making. It uses historical data and patterns to anticipate future outcomes. By analyzing vast datasets, it identifies underlying trends and correlations that might not be immediately apparent. This allows businesses and individuals to make proactive choices, backed by data-driven insights, rather than merely reacting to events as they unfold.
But how does this all work? Let’s break it down together.
The Science Behind Predictive AI
In essence, predictive AI employs algorithms that use statistical techniques (think regression analysis) to identify trends in massive datasets. Once these patterns are found, they’re used as predictors for future happenings – kind of like seeing breadcrumbs leading you toward an outcome.
Imagine being able to predict stock market trends with more accuracy than ever before because your system learned from past data points.
Predictive vs Reactive: The Key Difference
The real strength of predictive AI lies in its ability to shift businesses from reactive modes—where actions are taken after events have occurred—to proactive stances where decisions can be made based on anticipated outcomes.
To give some context, consider weather forecasting – once reliant solely upon human observation and experience now vastly improved by sophisticated models powered by…you guessed it right -predictive AI.
A Peek Into the Applications
You’ll find examples of predictive analytics across diverse industries—from finance predicting loan defaults or insurance claims risks; healthcare determining patient readmission rates or disease outbreak probabilities; retail optimizing pricing strategies or managing inventory levels—and the list goes on.
Have you thought about how predictive AI can revolutionize sales and marketing? Predictive AI is a game-changer here too. Ever wondered how some ads seem to read your mind, suggesting products you didn’t even know you needed? Well, that’s predictive AI in action, helping businesses target their audiences more effectively. Talk about customer service.
Data: The Fuel for Predictive AI
Data isn’t just about volume; it’s about quality. Accurate and relevant data fuels predictive AI, ensuring reliable forecasts. However, it’s not just about collecting data but continuously updating it.
As the world evolves, so should our datasets to keep predictions sharp. But with great data comes great responsibility. In this digital age, ensuring data privacy and ethical handling is paramount. In essence, data is predictive AI’s most valuable asset, driving informed and successful decisions.
Benefits of using Predictive AI
By offering unparalleled forecasting capabilities, predictive AI equips enterprises with insights that drive success. This is not just another analytical tool; Predictive AI is a strategic ally, guiding businesses toward a prosperous future.
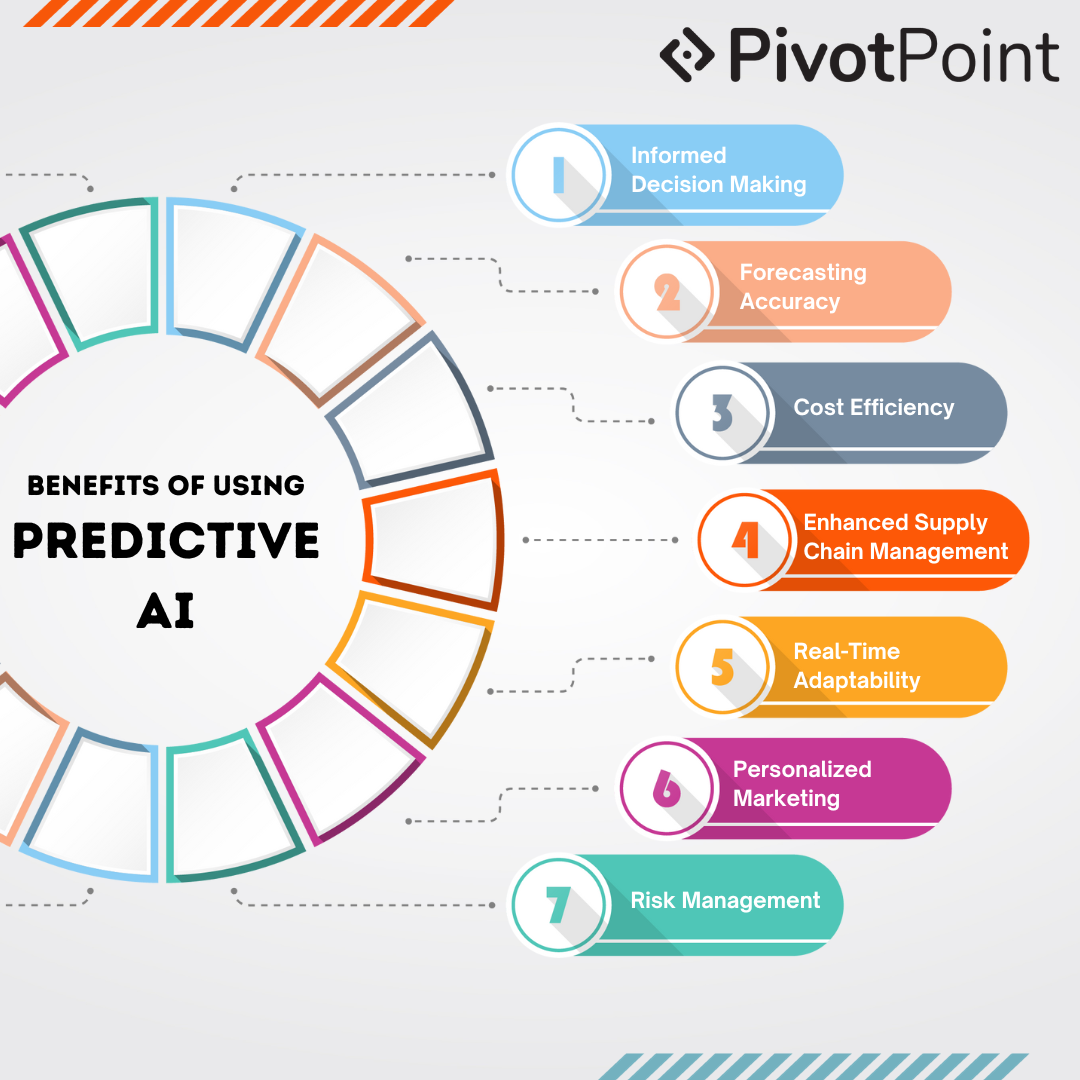
- Informed Decision Making: Predictive AI eliminates the guesswork by providing actionable insights based on historical data, allowing businesses to make well-informed decisions.
- Forecasting Accuracy: With the ability to analyze vast datasets, predictive AI can forecast future trends, such as stock market movements or consumer behavior, with remarkable precision.
- Proactive Business Strategy: Instead of reacting to events after they’ve occurred, businesses can adopt a proactive approach. Predictive AI enables companies to anticipate market changes, customer preferences, and potential challenges.
- Cost Efficiency: By predicting trends like consumer behavior or potential loan defaults, businesses can optimize their strategies, leading to reduced operational costs and increased profitability.
- Enhanced Supply Chain Management: Predictive analytics, when paired with AI, can optimize inventory levels, improve delivery times, and foresee potential disruptions, ensuring a smooth supply chain process and accurate levels of inventory management.
- Real-time Adaptability: Events like natural disasters, economic shocks, or global crises can disrupt predictions based on historical data. However, predictive AI can adapt in real-time, adjusting its forecasts based on current events and fresh data.
- Personalized Marketing: Predictive AI can analyze consumer behavior to tailor marketing strategies, ensuring that ads and promotions resonate with the target audience. This leads to increased engagement and conversion rates.
- Risk Management: In sectors like finance and insurance, predictive AI can anticipate potential risks, such as loan defaults or insurance claims, allowing businesses to take preventive measures.
Incorporating predictive AI into business operations can revolutionize processes, offering a competitive edge in today’s data-driven world.
Key Thought:
Predictive AI is a game-changer, using historical data and patterns to anticipate future outcomes. It’s like having breadcrumbs leading you towards an outcome. With predictive AI, businesses can shift from reacting after events occur to making decisions based on anticipated results. Whether it’s predicting loan defaults or optimizing marketing strategies – Predictive AI has diverse applications across industries.

Key Differences Between Generative AI and Predictive AI
Diving further into the AI realm, it’s essential to pinpoint the nuances that distinguish generative AI from predictive AI. Both have their spotlight in the AI theater, but when placed side by side, their unique attributes and applications become even more pronounced. Let’s navigate these distinctions and shed light on their individual strengths and weaknesses.
Generative AI: The Creative Whiz
In the simplest terms, generative AI uses algorithms to create new data from existing sets. It learns patterns in data and then produces original content that mirrors those patterns—much like an artist studying landscapes before painting one themselves.
This type of technology powers creative solutions such as writing songs or generating images from descriptions. However, it isn’t just about fun and games; businesses use generative models to develop product designs or generate synthetic datasets when real ones are unavailable.
Predictive AI: The Crystal Ball
Moving on to its counterpart, predictive AI, this form utilizes statistical techniques like machine learning algorithms to predict future outcomes based on historical data—it’s akin to looking into a crystal ball armed with facts instead of mystic energy.
Predictive models help marketers forecast consumer behavior or market responses by analyzing past trends—a priceless advantage in business strategy development where predicting customer needs can be game-changing.
Different Strokes for Different Folks
- Creativity vs. Prediction: While both forms leverage large volumes of data, they differ in purpose. Generative AI aims to create new data or content, while predictive AI uses existing data to forecast future events.
- Application: Generative models are useful for tasks that require innovation—like product design or creating synthetic datasets. On the other hand, predictive models shine in situations requiring foresight and planning like sales forecasting or market trend analysis.
Remember, it’s not about picking sides between generative and predictive AI. Instead, it’s about understanding their unique strengths and harnessing them effectively. By recognizing their distinct capabilities and integrating them where they fit best, businesses can unlock new potentials and stay ahead in the competitive market.
Key Thought:
Generative vs. Predictive AI: Generative AI crafts new data from existing sets, powering creative solutions like songwriting or image generation. It’s also used in business for product design and creating synthetic datasets. Predictive AI uses past trends to forecast future events, helping marketers predict consumer behavior and aiding in strategic planning.
Challenges and Considerations
As with any technological advancement, the application of both generative AI and predictive AI models comes with their unique set of challenges. Ethical concerns top the list for both types, while issues around data governance, security, and privacy also need careful consideration.
Ethical Concerns Surrounding Generative AI
Generative AI’s ability to create content that is almost indistinguishable from human-generated material raises a slew of ethical questions. There are worries about “deepfakes”—hyper-realistic digital forgery created using machine learning—that can be used to spread misinformation or conduct fraud. The line between creativity and plagiarism becomes blurred when an algorithm produces work strikingly similar to existing creations.
This isn’t just theoretical either; we’ve already seen examples in news reporting, where bots have been used to generate articles en masse. These concerns ask us: How do we maintain authenticity in a world where machines mimic humans so well?
Ethical Concerns Surrounding Predictive AI
Predictive AI relies heavily on data mining techniques which can infringe upon individual privacy rights if not handled correctly. For instance, imagine your buying habits being predicted by AI algorithms which then dictate what ads you see online—it feels invasive, doesn’t it? Additionally, there’s always the risk that predictions made could be incorrect leading to unfair consequences for individuals involved.
Studies show how biased training data sets may lead these systems into making prejudiced forecasts—a concern echoed across multiple sectors including healthcare, law enforcement, and finance.
Data Governance, Security, and Privacy Considerations
With AI models handling massive amounts of data, having strong governance is key. This involves making sure all data is obtained ethically and used wisely. To ensure ethical data use, businesses should evaluate the source of their AI-utilized data. Is its use compliant with regulations such as the General Data Protection Regulation (GDPR)?
Furthermore, businesses must ensure that personal and sensitive information is anonymized and encrypted. Transparency with stakeholders about how data is used, stored, and protected is crucial. Lastly, regular audits and reviews should be conducted to ensure ongoing compliance and to address any potential vulnerabilities in the system.
Key Thought:
Embracing generative and predictive AI brings unique challenges. Ethical issues, like the creation of deepfakes or infringing on privacy with data mining, need careful attention. Additionally, concerns about data governance, security, and privacy are crucial for businesses to consider when implementing these technologies.

Future Outlook
The convergence of generative and predictive AI promises to revolutionize how businesses forecast trends, generate synthetic data, and simulate various scenarios. But it’s not just about what these two types of AI can do separately—it’s the combined potential that truly excites.
Generative models, known for their creative prowess, could let us dream up new product designs or innovative marketing strategies. Imagine an algorithm that uses customer behavior patterns to craft the perfect ad campaign tailored to your audience.
Synthetic Data Generation: A Game-Changer?
In today’s world where data is king, generating synthetic data holds immense promise. Synthetic data has been a game-changer in sectors like healthcare and finance where privacy issues often limit access to real-world datasets. This artificial but realistic dataset allows companies to test algorithms without violating any ethical norms.
As we move forward, combining generative AI with machine learning techniques could enable even more nuanced simulations – giving rise to unprecedented opportunities for innovation across all industries.
Predictive Models’ Future Role
Moving over from creating things anew, let’s look at prediction models which excel at forecasting future events based on past behaviors or trends. We’re talking sales forecasts refined down almost individually – making sure you never miss a beat when it comes to understanding your market demand or response rate.
But there are plans for these predictive models to go beyond just forecasting. For instance, they could be used for personalized marketing—tailoring offers and ads to individual consumers based on their past behavior.
The Symbiotic Relationship Between Generative and Predictive AIs
When used together, generative and predictive AI models create a symbiotic relationship that can supercharge business strategies. Generative AI fuels innovation while predictive AI ensures those innovations are in line with market trends and consumer demands.
Consider Adobe’s Creative Cloud. It uses both models to help designers: generative algorithms generate fresh design ideas; predictive analytics ensure the designs meet current market tastes. The result? Designers get to produce work that’s not only innovative but also relevant.
Conclusion
Peeling back the layers of AI, we’ve seen two distinct yet powerful players – generative AI and predictive AI.
Generative AI, is your go-to for creative innovations. It’s capable of dreaming up new ideas and helping businesses think outside the box.
Predictive AI is all about precision forecasting. From sales trends to customer behaviors, it has an uncanny knack for spotting patterns that can fuel growth strategies.
Yet it’s not a question of ‘generative AI vs. predictive AI’. These twins are most potent when used together in business strategies – one sparks innovation while the other anticipates market responses to these novelties.
FAQs
What is the difference between predictive AI and generative AI?
Predictive AI forecasts future events based on historical data, while generative AI creates new content from learned patterns.
How does Generative AI work in marketing?
Generative AI systems are all about creativity and innovation. It generates content for campaigns or creates product descriptions based on current data inputs. IBM Research suggests it could even produce original music for adverts. Think of it as an imaginative partner that never sleeps. AI technology is producing fresh powerful forms of content 24/7. Generative AI offers the creative process tailored experiences and is known to personalize experiences better than a human.
As these generative AI companies advance, we’ll see more machines try to mimic styles of human interaction. We’ll watch product design generative AI analyze heaps of data to make improved decision-making predictive.
What role does Predictive AI play in sales forecasting?
Predictive AI uses historical data to make educated guesses about future outcomes. In terms of sales forecasting, this means analyzing past trends to predict future demand. Salesforce Einstein demonstrates how these accurate predictions allow businesses to prepare more effectively.
AI systems are changing business outcomes with the way they analyze data and analyze patterns to create statistical algorithms with greater accuracy. Being able to output realistic AI examples of a marketing strategy to improve customer conversations for example is valuable to any company.
Can I use both Generative and Predictive AIs together?
Absolutely. They complement each other perfectly; while one generates ideas, the other predicts their potential success. BuiltIn writes extensively on how they operate symbiotically within business strategies.
What are some ethical considerations when implementing these models?
Data privacy should always be a priority when using any form of AI, especially with predictive systems that analyze personal information. Generative AI can prompt qualms concerning originality and the protection of intellectual property.
How will Generative and Predictive AIs evolve in the future?
Traditional machine learning is great, but the possibilities for decision-making predictive AI to help inform business decisions and recommend products will undoubtedly enhance businesses all over the world. From synthetic data generation to advanced forecasting methods, both models have significant potential for growth. Personalization generative AI algorithms creating content tailored to the end users is pretty cool tech too.